In the Nature Research Journal, a review about Nature Machine Intelligence by J. Jiménez-Luna, F. Grisoni and G. Schneider, dealing with the central concepts of explainable AI (XAI) in the emerging field of Drug Discovery, underlines that various concepts of ‘artificial intelligence’ (AI) have been successfully adopted for computer-assisted drug discovery in the past few years. This advance is mostly owed to the ability of deep learning algorithms, that is, artificial neural networks with multiple processing layers, to model complex nonlinear input–output relationships, and perform pattern recognition and feature extraction from low-level data representations. Certain deep learning models have been shown to match or even exceed the performance of the familiar existing machine learning and quantitative structure–activity relationship (QSAR) methods for drug discovery. Moreover, deep learning has boosted the potential and broadened the applicability of computer-assisted discovery, for example, in molecular design, chemical synthesis planning, protein structure prediction and macromolecular target identification.
There are many domain-specific challenges for future AI-assisted drug discovery, such as the data representation fed to said approaches. In contrast to many other areas in which deep learning has been shown to excel, such as natural language processing and image recognition, there is no naturally applicable, complete, ‘raw’ molecular representation.
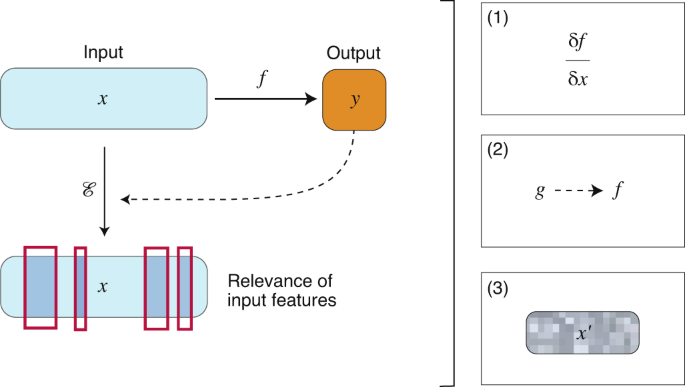
Drug design is not straightforward. It distinguishes itself from clear-cut engineering by the presence of error, nonlinearity and seemingly random events.
Designing new drugs epitomizes in the question whether pharmacological activity (‘function’) can be deduced from the molecular structure, and which elements of such structure are relevant. Multi-objective design poses additional challenges and sometimes ill-posed problems, resulting in molecular structures that too often represent compromise solutions. The practical approach aims to limit the number of syntheses and assays needed to find and optimize new hit and lead compounds, especially when elaborate and expensive tests are performed.
Click here to read the full article.